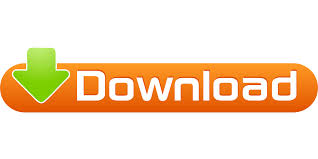
#Super resolution algorithm software
There are more than 30 software packages used for SMLM by the community, each varying in accessibility and processing power which have been subjected to quantitative analysis by studies such as the one by Sage and colleagues (2015). Choices made in both pre- and post-processing make a big difference to the image output quality, changing important factors such as the signal-to-noise ratio, detection accuracy, and subpixel fluorophore localization. This software processing can also apply to single-molecule localization microscopy (SMLM), where analysis can be vital in order to optimize data output. This processing requires specialized algorithms and analysis software, which must be capable of dealing with the large datasets of thousands of frames from 3D, fast-moving dynamic samples with hundreds of data points of varying densities. Finally, we perform iSMORE on both MRI and two-photon fluorescence microscopy, which demonstrates its generalizability.Some super-resolution data cannot be visualized directly, only after images are reconstructed and processed can they be displayed, such as in PALM and STORM. The third contribution is the use of an edge-based loss function and noise reduction to enhance the performance. The second contribution is that it uses a 3D network to preserve slice consistency. This iterative framework improves training data from thick slices to thinner slices after each iteration, thus improves super-resolution accuracy after each iteration, and solves the first issue. We show in this paper that one of the existing 2D CNN-based self super-resolution methods, SMORE, can be further improved by iteratively applying it using 2D or 3D networks, yielding 2D and 3D iSMORE. Regarding both issues as well as the generalizability of algorithm, we made four contributions. The second one relates to the 2D CNNs used on 3D volume, which cannot guarantee slice consistency. Training on thick slices is equivalent to training on averaged true HR images, which is suboptimal. The first one is that the assumption of HR in-plane slices is actually not solid since these thick in-plane slices are averaged true HR thin slices. However, there are two issues with these approaches. By degrading them into LR images, 2D CNNs can be trained and then used to restore the images in the through-plane. These self super-resolution methods assume that the in-plane slices are HR, and can therefore be used as HR training data. Since these data are often unavailable in practice, self super-resolution algorithms that do not need external training data have also been proposed.

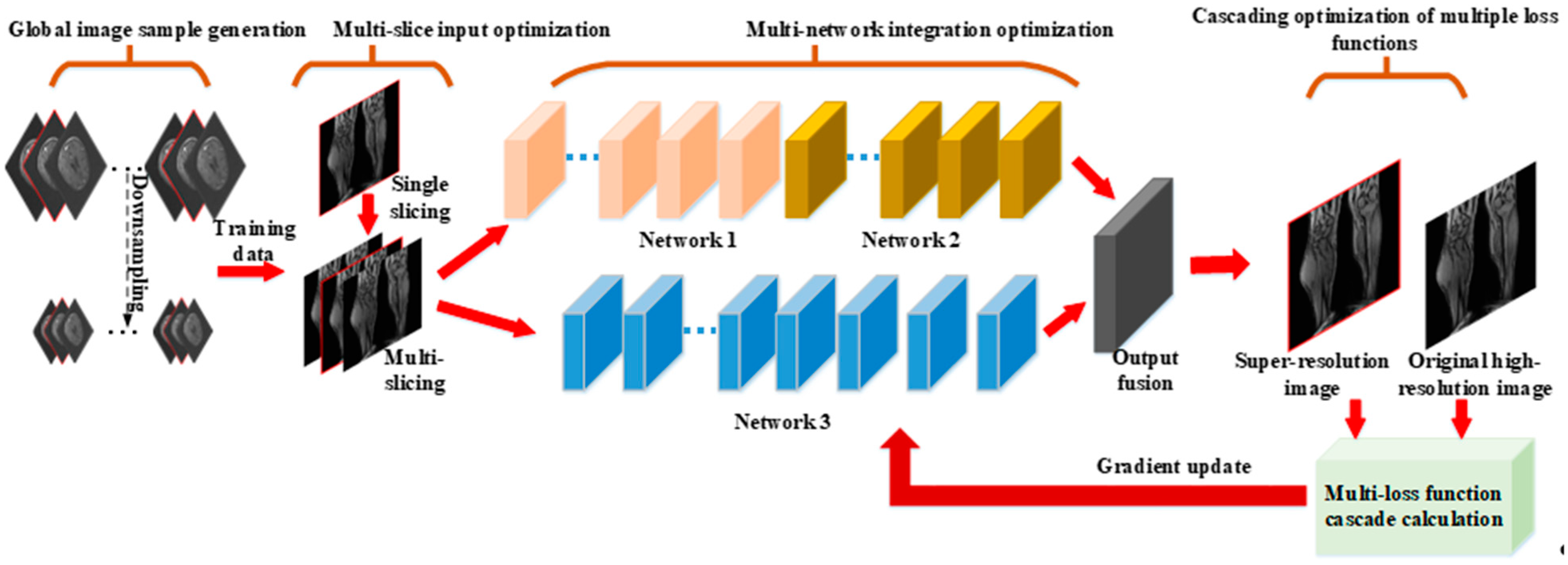
Instead, many researchers have proposed super-resolution algorithms including state-of-art convolutional neural network (CNN)-based methods that require matched training data that have paired LR/HR examples.
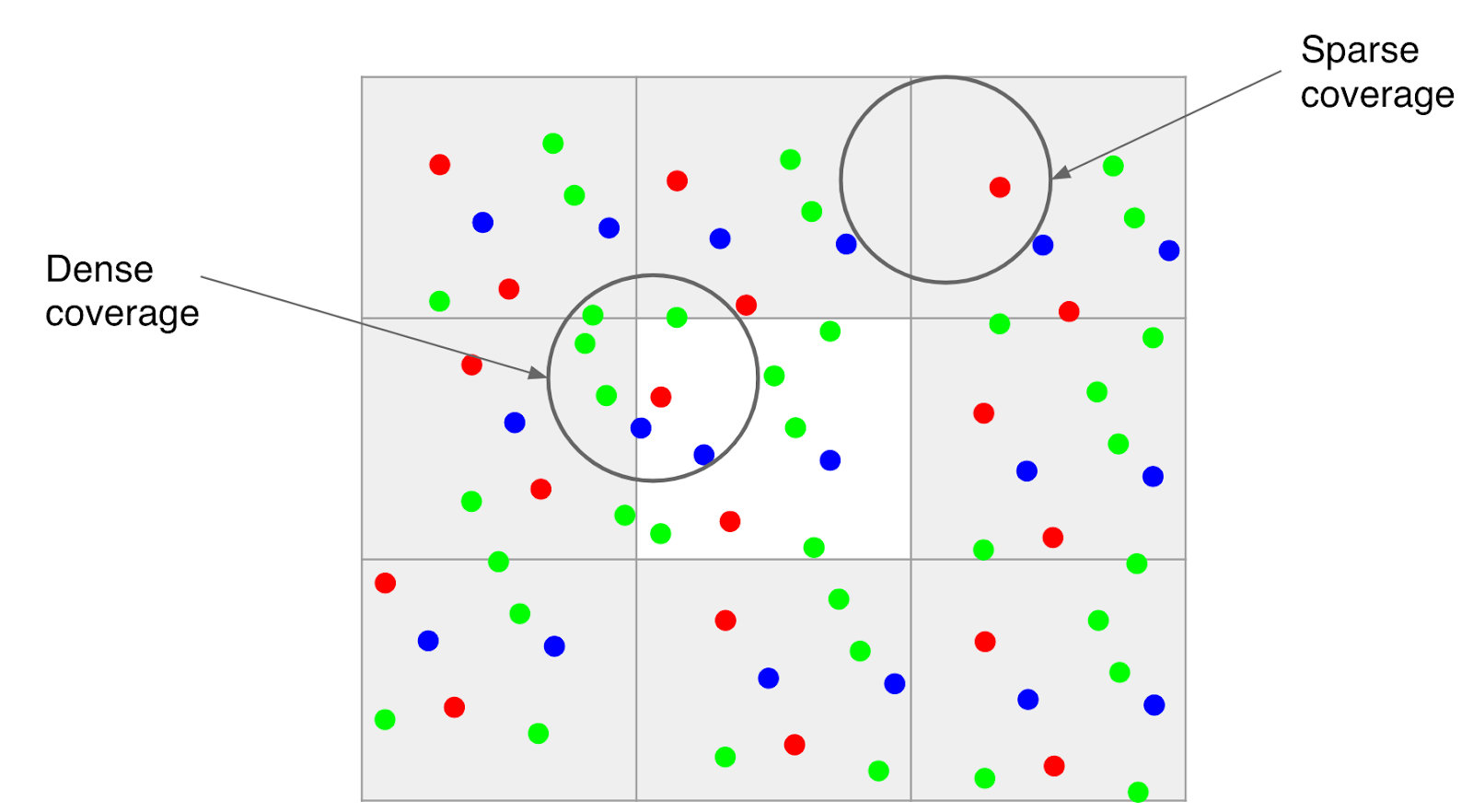

The blurriness and aliasing artifacts that result cannot be solved by simple interpolation. In practice, however, many acquired images, including magnetic resonance imaging (MRI) and fluorescence microscopy, have HR in the in-plane directions and low resolution (LR) in the through-plane direction. In 3D medical imaging, images with isotropic high resolution (HR) are almost always preferred.
#Super resolution algorithm series
Book series (LNCS, volume 11827) Abstract
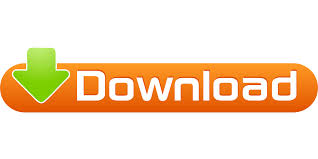